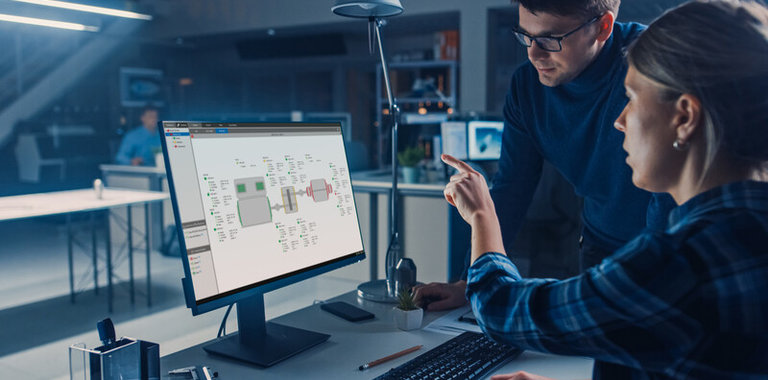
Predictive Maintenance Overview: The Natural Result of a Condition Monitoring Strategy
As any experienced plant operator will tell you, your machinery assets are never constant. You know that even if only on a microscopic level, your best machinery assets are, in fact, deteriorating towards an eventual failure. Fortunately, the countermeasures we employ in this industry are constantly evolving to make our job of managing those critical assets easier, and more importantly, more effective.
One of the most exciting and promising areas of improvement is in the Predictive Maintenance(PdM) space. Not only is this a natural progression from your typical condition monitoring strategy, but it brings with it numerous benefits that make the life of an asset manager/plant operator that much easier - to say nothing of the operational benefits PdM can bring.
But first, let’s get a firm understanding of PdM.
While the subject of predictive maintenance may be relatively straightforward for some, when discussing this topic we’ve found that the terminology can get confused or misused, so let us start with some definitions. First let’s start with the more familiar form of maintenance, Preventative Maintenance.
Preventative Maintenance is defined as maintenance that is performed according to a fixed schedule, or according to a prescribed criterion, that detects or prevents degradation of a functional structure, system, or component, in order to sustain or extend its useful life (ISO 13372).
A classic example given for this maintenance strategy would be changing oil and filters every X many miles or hours on an engine. This is done not because the filters have been found to be blocked or because of poor oil condition but because it is believed that the components, if changed at that interval, will still be within their useful life, resulting in the machine avoiding a functional failure before it manifests any symptoms.
This expected point of component degradation is often based on recommendations from the equipment OEM and that information is often derived from empirical study, simulations, and experience gained from reviewing data from their fleet of similar machines.
So with that understanding, let’s discuss Predictive Maintenance, and how it differs from Preventative Maintenance.
Preventive maintenance can be thought of as a maintenance strategy. It is not a maintenance strategy for all, however. Depending on the criticality of the machinery in question, it may or may not be the most appropriate strategy for that machine and your maintenance budget. Another example of a maintenance strategy can include run to failure, which given the relevant machine/component at a particular criticality can also be a very valid approach.
Predictive Maintenance is often defined as a maintenance strategy that is based on monitoring and measuring the condition (i.e. condition monitoring) of the assets to determine whether they will fail during some future period and then taking appropriate action to avoid the consequences of that failure. This approach is often still employed for more critical machinery, and for a long time this approach was mostly used on only the most critical assets. However, the advantages of a predictive maintenance strategy can today make it an appealing choice for many other less-critical machines as well.
For a while these machines may have been deemed “less-critical” traditionally because of their lower replacement costs or seen as ‘auxiliary systems’, but when they underperform, they can still have a serious impact on your critical processes. The advantages of a PdM approach include reducing waste and unnecessary downtime as it has the potential to make maintenance activities more focused and has the added benefit of being able to offer developing an understanding of your machinery through data collection and interpretation. One such area of waste that PdM helps resolve is the area of over-maintenance. With an accurate dataset and real-time condition status, you won’t have to rely on bi-weekly visual inspections; your data will speak for itself, thus allowing you to elsewhere allocate those resources that would have been spent on inspecting and maintaining an asset that didn’t need it.
To illustrate the differences let’s consider our oil and filter change from before. In the predictive maintenance approach, we would monitor the differential pressure across the filter to check for excessive blockage in the filters and we could monitor particulates in the oil or review its viscosity, water dilution, or TAN index to help us determine if the oil requires changing. Not only does this give the potential in cost savings by reducing ‘unnecessary’ oil changes but also provides more monitoring and data which can often catch emerging issues that manifest as deviations in the condition of the oil system for that machine. A true win/win.
So given that the definition of predictive maintenance requires a strategy that is based upon monitoring and measuring the condition of the assets, it will come as no surprise that condition monitoring, which can be defined as the acquisition and processing of information and data that indicate the state of a machine over time, forms an integral part of any predictive maintenance program.
Condition monitoring can be viewed as one tool that, when used effectively, can be part of a successful predictive maintenance program. However, a successful predictive maintenance program will require quite a bit more than just good instrumentation, data analysis, and the reporting of faults. It needs a repeatable process visible at all levels of the team(s) supporting the program that ‘closes the loop’ on every case or issue identified and drives that case to conclusion and records its outcome thus allowing the program as a whole to continually improve over time.
This type workflow (see below) is something that will be familiar to many, however, implementing good case management to effectively support a PdM program is a critical and often challenging part of the process. However, it is also a topic deserving its own blog. In short it is an area that has seen and is set to see in the near future ever improving software and tools produced to address the needs of this way of working; particularly in regards of making use of the latest AI and data replication tools available.
The concept of monitoring a machine’s condition and only conducting maintenance to that machine once a change in the condition of the machine is detected is not new. Some of the roadblocks to a truly predictive maintenance program have been:
- Costly instrumentation/computing power (per machine)
- Data siloed, lack of interconnection (moving people not data)
- Costly data storage
Today, instrumentation is cheaper and more accessible than ever. Consider that we live in a world where a TB of cloud storage costs less per month than a coffee order and the same can be said of the computing power to accompany it. Data interconnection is possible to such an extent nowadays that it brings with it its own set of new challenges: cybersecurity, data sovereignty, and simply having the human resources to review and realize the value from all of the data now available.
We’ll discuss how preventative maintenance is now just a component of a larger umbrella of predictive maintenance. We’ll also show how preventative maintenance can effectively guard you against issues but how it may also mean you are over-committing resources by applying blanket rules to your assets, thus wasting resources and cutting into your profitability through unnecessary maintenance.
Monitoring the condition of machinery to help determine the maintenance needed is no longer the direction of the near future; it is in some cases not even the direction of the here and now, but the recent past. What we are looking towards now builds on the progress made through the correct (and incorrect) adoption of various condition monitoring programs. It looks to address the new challenges posed by the sheer mass of condition monitoring data collected, how to effectively triage it, and provide the tools needed to have effective maintenance.
Condition Monitoring is the essential, underlying “governing dynamics” of a successful predictive maintenance strategy. Any prediction, be it weather, sporting event outcomes, or asset management all require the same fuel: data. Your predictive maintenance outcomes are only as good as the data you can feed it. So, to get the best, cleanest, most actionable data, you need sound policies and products in place.
As with any manifold solution, you need a level of unification. If your datasets are incompatible, you open the process up for reliance on time intensive human interpretation and tuning requiring human resource that you often do not have or wanted to reduce by adopting an AI solution initially. Then there is the cost of scale, if your systems don’t seamlessly integrate, how can you grow at a satisfactory (read: affordable/ROI-approved) level? By leveraging the ability to unify through single-source solutions, BN ecosystems, as well as added support services such as HOST, etc… you can achieve an economy of scale by reducing the scale of your reliance upon multiple vendors, thus allowing your existing technicians the ability to manage a larger system, and thus focus on far more critical matters versus less critical maintenance issues. Savings, savings, savings.
And even though we could go on and on about how our solutions for attaining a predictive maintenance strategy are amazing, we find it better to let our partners do the talking. Here are a couple of case studies where our customers came to us for help in making the jump from preventative to predictive maintenance, and the benefits they attained:
Case Study/Use Case: Bently HOST: Achieving Peace-of-mind through an Outcome Focused Solution
It comes down to what you want to accomplish and how you plan to go about obtaining your desired results.
At Bently Nevada, we view ourselves as a partner with all of our customers, from the initial point of contact to relationships over sixty years in the making. We pride ourselves on helping our customers find solutions to their condition monitoring and predictive maintenance needs while earning our place as the industrial world’s leading domain expert and solutions provider in this space.
To that end, we invite you to contact us to see how we can help take your condition monitoring strategy from reactive to predictive. We’re confident that the right combination of our products and services - backed by our industry-leading domain expertise - can improve your asset management strategy.