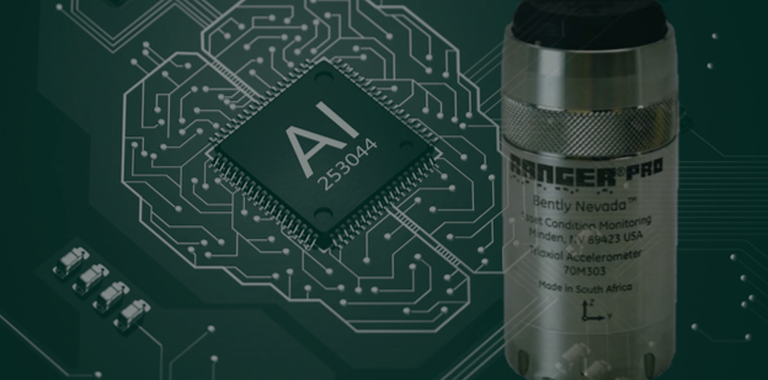
When Ranger Pro meets AI, good things happen
Introduction
Today, your Ranger Pro installation brings data into System 1 where you can trend it, view it, alarm on it, and even send detailed notifications to appropriate personnel while launching workflows with our newly released System 1 APM1 product. And if you've installed System 1 at an enterprise level, you have visibility across your entire fleet of machines - no matter your mix of data acquisition strategies: wired, wireless, or portable.
But there's a catch. You have to set and maintain those alarms. You have to review that data. You have to analyze that data to figure out root cause and remedies. While there is nothing wrong with this approach, truth be told, most customers find they are stretched thin and could use extra help. Even those customers with the resident skills to turn data into action are finding that they simply don't have time and are looking for any available means to improve efficiencies.
The real question, then, isn't usually whether you need help. It's what kind of help you should employ. This is where AI enters the picture. Yes, I said AI. However, before you roll your eyes and push this article to the side, let me acknowledge that AI has left a bitter taste in many mouths by over-promising and underdelivering. In fact, nearly half2 of the customers we recently polled expressed hesitation about using AI when it came to machinery condition. So, I'll make a big ask here: give me the benefit of the doubt and keep reading for the next few minutes. I want to directly address the issue of AI-skepticism in this article rather than pretend it doesn't exist by answering some fundamental questions:
- Can you really trust AI with machinery analytic applications or are they more hype than substance?
- Where is the right place to apply it, and why?
- What problems does it solve?
- Is it AI versus people or AI plus people?
Spoiler alert: AI not only works, it works exceptionally well - when you harness it correctly.
AI - an evolution
The focus of this article is not an exhaustive recounting of Bently Nevada's journey with so-called "expert systems" and AI. A companion blog by Hayley Stephenson addresses that topic in depth and shows how we have evolved over the years. Suffice to say here that Bently Nevada was a pioneer in this space with introduction of our Engineer Assist software in 1992. Since that time, we have continuously offered various types of first-principles, physics-based solutions along with the more recent addition of true AI (data science-based rather than physics-based) technology.
In a moment, I will address the above list of AI questions directly. First, however, I'd like to look at what class of assets were historically addressed by AI / Expert Systems and why that is changing. Then, I'll dive into what makes today's AI such a good fit to address industry's most pressing problems on a different class of assets.
Target assets
Industry has historically applied AI and physics-based expert systems to only their most critical machinery. The reason is quite simple: critical assets typically represent the biggest risk and warrant the best instrumentation. It's a no-brainer to monitor these assets.
Today, customers are telling us quite clearly that although critical assets may have been the most important reliability improvement candidates decades ago, that is no longer the case. Industry has indeed spent the last several decades addressing reliability issues on those assets. As a result, they are typically today the best instrumented and most reliable assets in the plant - often running for years at a time between planned outages. They were addressed first precisely because the stakes are so high with respect to downtime, safety, environment, and costs of repair.
But with that task largely competed for most customers - particularly in the continuous process industries like petrochemicals and electric power generation - industry is turning to another class of assets: those deemed less-critical.
The "many versus few" factor
The reality is that in most plants, there are only a few critical assets. In a refinery, for example, the ratio of assets in the critical category to those in all other categories is about 1:100 (see sidebar for details). In other industries, the ratios vary, but the basic rule holds true: "the critical few" are vastly outnumbered by "the less-critical many". Thus, where conventional wisdom has been that customers needed the most help with their critical assets, today - as noted above - their critical assets are in good shape. And, they don't require a veritable army of people to manage.
In contrast, customers today are having a much harder time managing the "less-critical many" because it becomes "death by a thousand cuts". Even if only 3% of their less-critical assets have issues, when there are ten thousand such assets, that's 300 screaming for attention at any given moment. Is the alarm real? Is it process-related or machinery-related? Is it urgent or can it wait? Thus, the sheer scale of less-critical assets creates a new set of problems that generally aren't present with the much smaller population of critical assets.
The "less-critical many" issue was underscored recently for us by the digital transformation leader of a large multi-national petrochemical manufacturer with a global footprint. Although each of their nearly 30 plants has dozens of critical machines, each has thousands of less-critical machines. This equated to more than 100,000 such machines across the enterprise. Because there are so many, and because they are often spared, not as much attention has been given to their reliability historically. It was reasoned that if a machine fails, there is a spare to take its place and the reliability of such assets was simply not as vital to the bottom line.
However, the digital transformation leader explained that this attitude does not address the direct and indirect costs of fixing so many assets. Nor does it fully recognize that the standby asset may have suffered a hidden failure that is not revealed until it is needed and fails to start or starts with obvious problems and cannot run. Nor does it address the fact that the primary asset may fail in such a way as to cause a toxic spill, a fire, or some other high-consequence issue that cannot be prevented simply by having a spare.
The example cited by this digital transformation leader - who incidentally has a very strong background in rotating machinery - was that such machines might be running with a Mean Time Between Failure (MTBF) of 18 months, but by increasing to 28 months this would translate to tens of millions of dollars in savings across the enterprise and would have a high ROI. It also meant that if he had essentially continuously gathered data that was not reliant on portable data collection intervals, the asset was far less likely to fail catastrophically. Intervention would occur before that could happen.
He understood that most companies - including his own - already addresses such assets with portable data collection strategies. However, as a digital transformation leader he was looking for step-change improvements, not just incremental improvements, and saw this class of assets as the single most fertile area for improvement with very high associated ROI. He was savvy enough to understand that simply putting IIoT sensors on all these assets was not going to be the solution, because he would then need a large team of people to analyze the incoming data. His vision was instead for AI to reliably analyze the incoming data and detect problems so a relatively small team could manage a very large population of assets. The team would thus be focused on correcting problems, not detecting problems and collecting data.
As a Ranger Pro user, you have already understood the importance of IIoT sensors and moving away from a strategy that was based solely on portable data collection. Until recently, however, what we have not been able to offer you is robust AI to assist you with those IIoT sensors. I'm pleased to announce, however, that is no longer the case.
1:100? Yup.
Why AI?
AI is all about leveraging your people to do the things that only people can do while relinquishing the tasks that no longer have to be done by humans. As a Ranger Pro user you've relinquished part of those tasks: data collection. Now it's time to consider another part of those tasks: data analysis. This frees you to do what machines cannot: fixing identified problems and eliminating machinery defects.
AI has now progressed to the point that it can detect anomalies with greater than 95% success. The particular AI that we have chosen to use for assets with rolling element bearings goes beyond just detecting that something is wrong and can tell what is wrong with 99% accuracy. I realize that sounds too good to be true, but across an installed base of more than 100,000 monitored machines spanning 300 million hours of runtime with this technology, those are the actual results.
The AI we are using in conjunction with Ranger Pro sensors does not require any training. It knows about pumps, motors, gears, fans, blowers, compressors, and more. It knows about rolling element bearings. About imbalance. Misalignment. Loose parts. Foundation problems. It even knows what a sensor fault looks like compared to legitimate machinery faults. When we baselined this technology against conventional approaches, it resulted in 60% fewer false positives and 20% fewer false negatives, meaning you don't spend time chasing non-existent problems - disassembling machines only to find no problem was there - and you don't have as many undetected problems that sneak through and only become apparent when the asset fails.
We don't use AI divorced from human experts, either.
When an anomaly is detected, it is validated by one of our team of human vibration experts who then adds context and recommendations such as corrective actions, severity, etc. This is then pushed to you in the form of a notification and you are provided with workflow tools as part of our web-based Machine Health platform. It allows you to open cases, assign tasks, follow up on issues, and "keep score" of how issues were ultimately dispositioned as part of a holistic reliability improvement process.
Machine Health as a Service
This offering is called Machine Health and it is delivered as a highly affordable subscription service based on number of machines – not number of measurement points. By transitioning your Ranger Pro installation to our Machine Health offering, we automatically assume responsibility for maintaining all your hardware and software infrastructure associated with Ranger Pro, offloading that burden and cost from you. We also provide the option of you adding additional ranger pros if a more complex machine is deemed under-instrumented to ensure comprehensive health can be addressed.
Your Ranger Pro data is sent to the cloud, analyzed using the powerful AI described above, and then advisories are delivered back to you.
Benefits
By enhancing your Ranger Pro infrastructure with our Machine Health offering, you obtain the following benefits:
Freedom from alarm management and manual data interpretation
Data is reviewed automatically and continuously while intelligent, human-vetted alarms are reduced by 99%. Alarms now take the form of "misalignment" "unbalance" "worn belt" "cavitation" "loose foundation" and the like instead of "high vibration" or "anomalous pattern detected".
Rapid Analytics / Prescriptive Diagnostics
The system begins working the instant we connect your data to our cloud-based AI. No waiting for the system to train itself. Anomalies are detected quickly, accurate AI diagnostics are rendered quickly, and human machinery experts review each and every finding before adding important context and recommendations. Results are then sent to you in the form of actionable notifications that can be addressed using the integrated workflow tools in our Machine Health offering.
Enterprise Scalability
Our Machine Health offering is highly scalable. More machines under monitoring no longer translates to more people. It simply translates to the deployment of more infrastructure (sensors / networks) - yet all of that infrastructure burden rests with us - greatly simplifying your role and even IT's role.
Accelerated Time-to-Value
Because the AI used in our Machine Health solution is purpose-built AI, it doesn't require so-called "training" and the need for extensive historical data before the system can begin providing valuable insights. In fact, insights typically occur within the first month and sometimes as quickly as the first 7 days.
Summary
As a senior product manager, instantly being able to deliver more value to customers like you simply by providing easy access to the power of AI is immensely gratifying. It really is like embedding a full-time machinery engineer to continuously review your data, spot issues, and deliver intelligent, prescriptive advisories.
Please contact us to learn more about enhancing your Ranger Pro and System 1 installation with our Machine Health solution, taking your plantwide and enterprise-wide asset health capabilities to the next level.
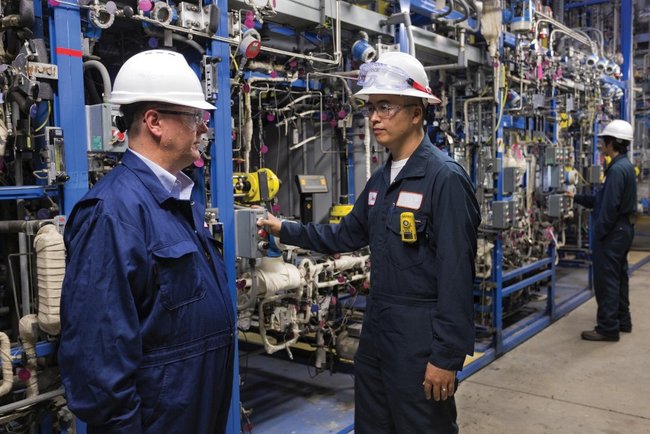
Real results. Extraordinary ROI.
One existing Ranger Pro customer that made the transition to Machine Health achieved the almost unbelievable: 25X ROI within 53 days. While we cannot promise the same results from every installation, 3X-10X in the first 6 months is typical. What do you have to lose by learning more? Reach out today and let us show you how to supercharge your Ranger Pro installation.
1Asset Performance Management - the linking of Asset Health Management, Asset Strategy Management, Asset Defect Elimination, and Asset Maintenance Execution (via an EAM system) under a single, unified environment.
2In a September 2022 poll of customers, 46% felt the promises of AI were "too good to be true" while 27% reported that their past experience with AI from other providers had been characterized by "overpromising and underdelivering".