Nikhil Gulati, Senior Director of Applied Machine Learning and Engineering at BHC3, enjoys thinking about what artificial intelligence (AI) could do when applied to the industrial realm.
‘’For example, an operator wants to optimize production across multiple fields and forecast production over the long term. Depending on the production profile and recovery methods, cost is also a factor to manage’’ says Gulati. ‘’Now add the need to track emissions in real time during production and potentially optimize with an additional constraint on emissions. This suddenly becomes a multi-dimensional and multi-objective problem to solve, from production planning, recovery to storage,’’ he adds. ‘’This requires more data, more granularity of the data, and a different approach to solving the problem. Current systems based on physics and rules embedded in a traditional software can’t solve for multiple objectives while keeping all the variables in play.’’
“My hope,” says Gulati, “is that in the next 10 years, that challenge will be solved by AI.” Among the many transformative outcomes of applying AI to the critical problem of how to bring cleaner sources of energy to market, is that the system will tell production engineers how to adjust production for the combined objectives and advise alternate forecasted scenarios. The engineer could then rely on that recommendation. We have seen similar multi-objective problems being solved for self-driving cars through a combination of decades old techniques in control systems and new techniques from AI. Just like in a self-driving car, you would need multiple algorithms to run at the same time and sensing at a completely different resolution, solving for all these objectives, and orchestrating a decision.

What’s getting in the way of more, scaled projects like this?
At the moment, says Gulati, people aren’t talking enough about outcomes, “not in the sense that I would like to see”. According to Gulati, they’re still talking about data and how to organize and leverage it for building a basic machine learning (ML) application that can run 24/7 reliably without breaking. ''I still hear strategy before outcome definition and quantification of value''. But the trend, he says, is to recognize that outcomes are paramount, and to collate and organize data in a way that allows it to be applied beyond silos of equipment efficiency, cost management or emissions control.
Unleashing the secret sauce
The second trend Gulati sees in industrial systems is towards an open ecosystem of expertise and software solutions. “One of the reasons other industries have flourished is because there’s so much open source,” he says. “Google builds something and open sources it, and then five other companies take it to a whole other level.” In comparison, oil and gas has been closed and proprietary about its intellectual property. But Gulati sees “a trend around AI and machine learning, where one company may solve a problem with compressors or turbomachinery and will either allow other people to build applications on top of it, or to add to an ecosystem of integrated solutions — an ecosystem like the Open AI Energy Initiative.
Launched in February this year, the initiative seeks to develop an environment of interoperable AI-based solutions for the energy and process industries. Founding members are Royal Dutch Shell, C3 AI, Baker Hughes and Microsoft. It has kicked off with an initial focus on Reliability and predictive maintenance solutions to improve operational efficiency.
“The idea is, instead of competing with each other, can we create an open ecosystem of all these products where we exchange information to create a sum of parts better than just individual capabilities?” asks Gulati.

Harnessing the benefits of big-picture analysis
How much more can be wrung from predictive maintenance solutions, which have now been applied for over a decade to critical machinery? “I think about this and talk with our customers about where the benefits are,” says Gulati. The point is that such solutions have so far been applied mostly to critical machinery, “But what if there’s a process problem that causes the equipment to go down?” The equipment itself may be fine. Gulati says the BHC3 Reliability vision will “span across processes, across different subsystems and take a holistic view of the reliability of an entire plant — that’s where the industry is moving. We’re going from analyzing one type of equipment to the whole process, understanding downstream and upstream process upsets which could cause equipment to come down.”
An engineer, turned scientist, turned machine learning leader, Gulati says his passion is applying technology to drive real business outcomes. He has moved in and out of academia, devoting himself to fundamental research on autonomous systems, adaptive wireless communications and more, and taken his lightbulb moments back into industry. Perhaps his real gift is in straddling the nexus between R&D and human understanding of how technology can be applied. “The people parts really draw me,” he says.
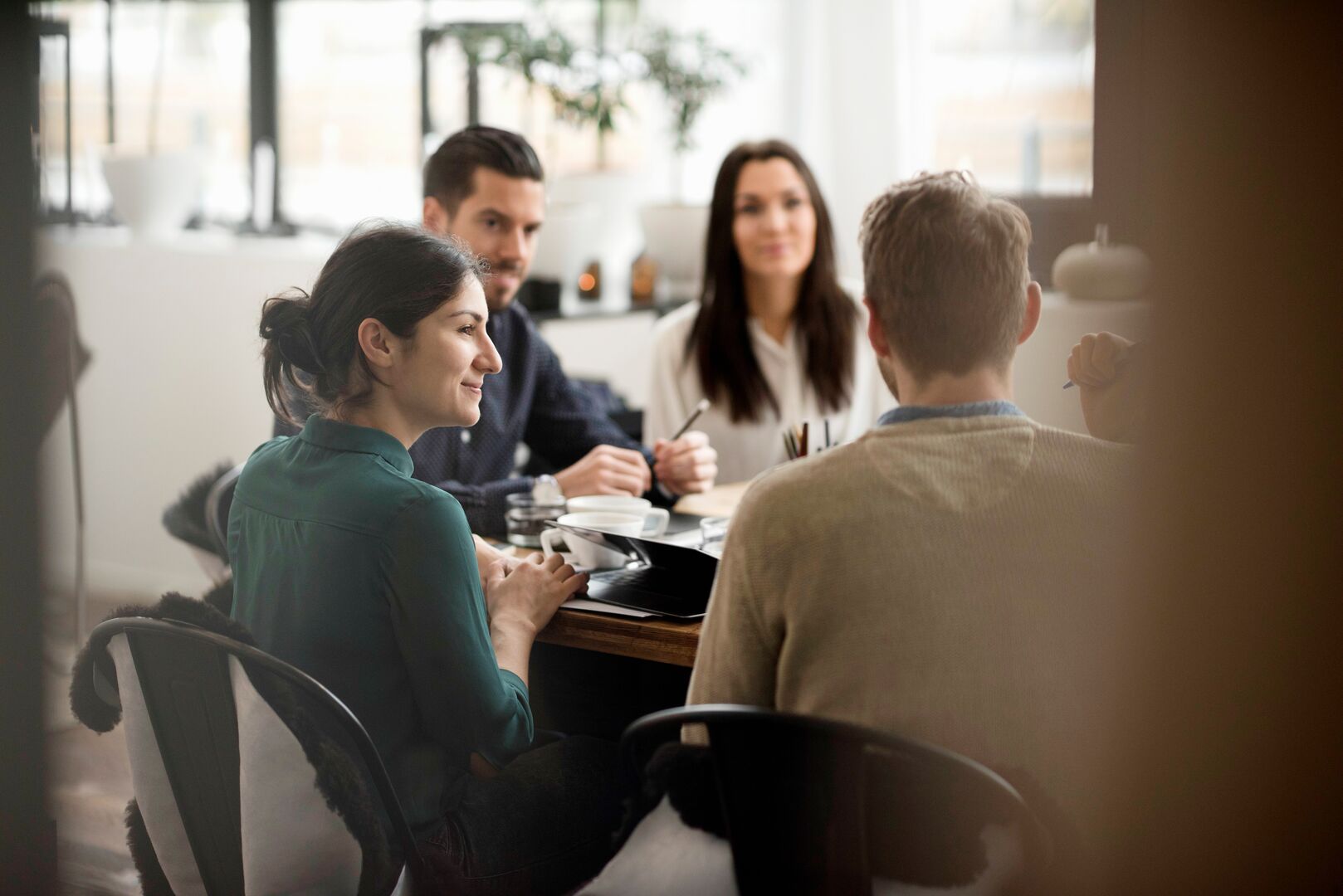
Fostering understanding at the nexus where people meet AI
On a business level, he says, “I love consensus building. There’s a lot of confusion in this new AI/ML space around what works and where the value is, so I like to bring together different thoughts and approaches to drive decisions.”
Gulati also motivates his talented teams towards common goals. “Technical people are very heads down, and I love that — that’s how they solve problems,” he begins. “But I also want to give them the right vision and put processes in place that enable them to do their best.” He says that projects fail where the vision isn’t clear and business impacts are not identified. There’s no point having a machine learning and data science team blue-skying it. “The business problems have to be defined for data scientists and a new breed of software engineers to shine.”
Baker Hughes is the industry partner and a major sponsor of the C3.ai Digital Transformation Institute, also established in February this year, which aims to advance AI for energy and climate security. Its opening gambit was to invite developers, researchers and scholars to submit proposals for research topics in areas such as: AI for carbon capture and storage; Cybersecurity of power and energy infrastructure; AI to increase the penetration and use of distributed renewables; AI for energy-efficient buildings and factories; AI to increase the safety and reduce emissions of hydrocarbon production and transportation infrastructure; and AI for improved climate change modelling.
Gulati’s role here is to identify research projects that complement Baker Hughes’ goals of moving energy forward in a carbon-minimizing world, helping the researchers to hone their goals, and supporting their efforts.
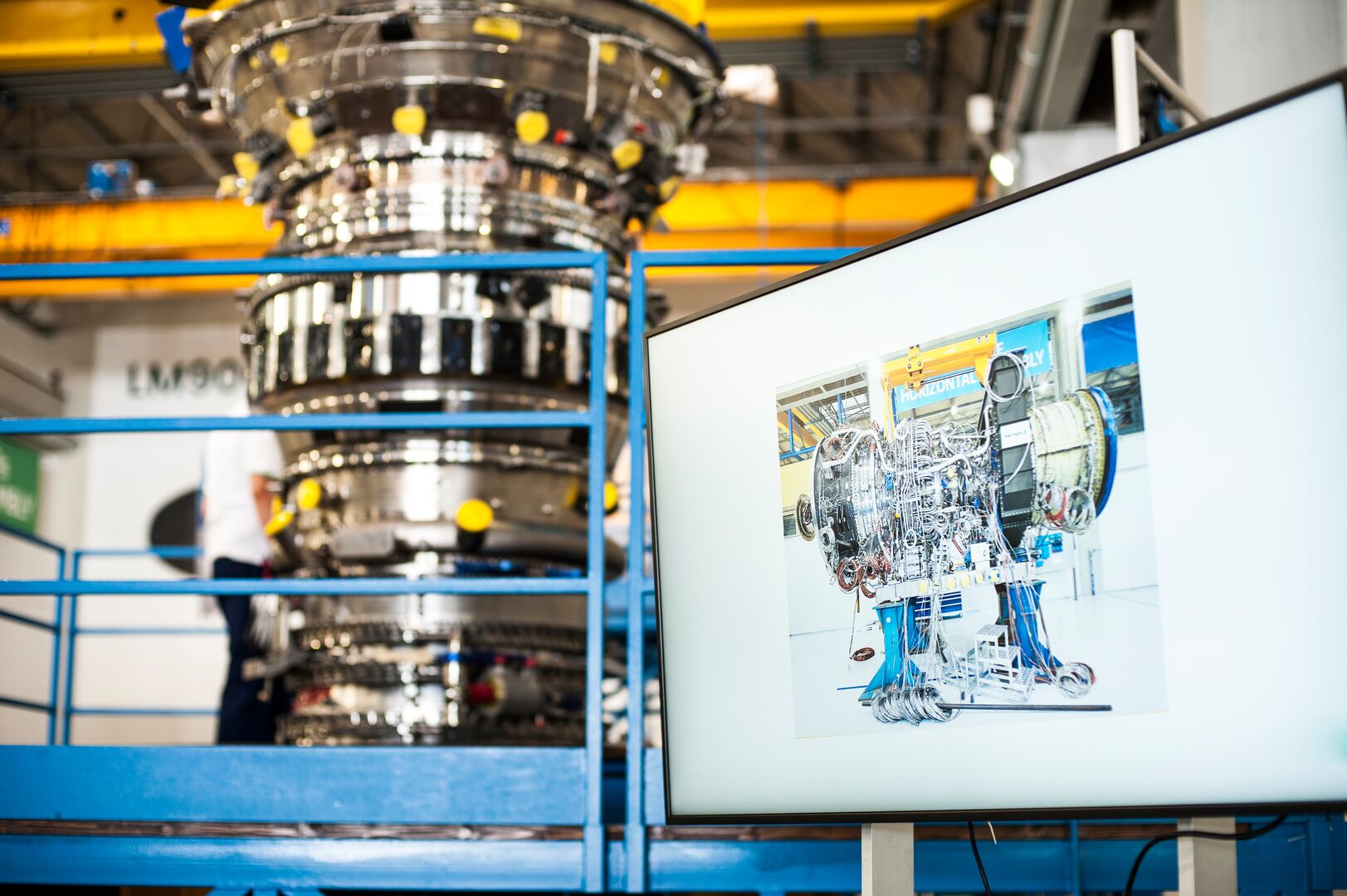
Is decarbonization and energy transition really working?
The scientist in him is also seeking quantitative analysis of how oil and gas-industry efforts to decarbonize and transition will positively impact climate change. “The line of dots has not yet been connected in my mind,” he says. Many companies have pledged to be carbon neutral by 2050, but Gulati says, “somebody has to bring data from somewhere to verify that and track the progress. We need to get better at quantifying our own emissions and help our customers do the same”.
He says his father is a local activist for social change and climate change mitigation in India, and Gulati Junior has told him that “The oil and gas industry has a bad rap, but I can’t just sit outside the industry and expect it to change — I’m going in!” He wants to be able to see where and by how much the industry’s efforts are shifting the dial.
Energy Forward Stories
Sign up to stay up to date on the latest innovations and people shaping the future of our industry.